Utilizing Advanced Analytics Capabilities to Respond to COVID-19
By Onur Torusoglu, VP, Chief Digital & Analytics Officer, St. Luke’s Health
The COVID-19 pandemic forced so many aspects of life to change and for health care to adapt in completely new ways. At St. Luke’s Health System, the need to adapt sparked innovations, many of which were driven by our Digital and Analytics organization. The St. Luke’s Advanced Analytics team, led by Dr. Justin Smith, developed COVID-19 modeling to help predict the virus’ path through our footprint and to optimize staffing. Our suite of Machine Learning and Artificial Intelligence (AI) models have helped shape our enterprise, providing insights towards safely and responsibly providing healthcare services during the COVID-19 pandemic.
Because the Rocky Mountains divide our coverage area, traditional susceptible, infected, recovered (SIR) models were not supplying meaningful results when forecasting the future surge of COVID-19. Our team developed a new SIR model that allowed us to adjust for the probability of social mixing between geographically distant populations – enabling us to accurately forecast our summer COVID wave within a week of reality. We also created simple but effective COVID regression models for forecasting hospital census up to two weeks into the future.
Perhaps our greatest advancement, though, came in a novel approach using Machine Learning and AI to project COVID patient hospitalizations – the XGBoost Model. This new model provides 30-day hospital and Intensive care forecasts that are accurate within +/- 4-5 patients across four hospitals and eight separate Intensive Care units, allowing operational decision-makers and executives to plan for future staffing and patient decisions. XGBoost was successful from early on despite such little data and more accurate than statewide models after accounting for Idaho’s unique geography.
The XGBoost Department Forecasting model is determined accurately by a validation method that retrospectively withholds actual results and creates predictions for those data points. The metric used to analyze the accuracy is Mean Absolute Error (MAE). MAE measures the average magnitude of the errors in a set of predictions without considering their direction. This model’s validation is designed to withhold 7-days of historical data and then project those same 7-days of historical data. The projections from the 7-days are then compared to the actual result and the MAE is then determined.
XGBoost is a highly sophisticated ensemble machine learning algorithm used to determine the individual projection for each day. This model’s XGBoost algorithm has been slightly shifted from the original XGBoost model and finds a continuous outcome versus a binary outcome. XGBoost follows a gradient boosted tree format that creates a hierarchy of predictive features that will filter down to create a projection. The XGBoost algorithm will create a gradient boosted tree algorithm specific for each projection day, and the output generated will be used and inputted into the three and ten day horizons. This means that an XGBoost model can be constructed to project every single projection directly across a 3-day horizon and 10-day horizon.
Regression Forecasting
The purpose of these forecasts is to supply a short-term projection for ICU and Adult Unit Hospital utilization, specifically for COVID-19 positive patients. The current projections are based on St. Luke’s data and are created using linear and polynomial regression. St. Luke’s Advance Analytics provides the two projections in an attempt to supply an outlook on short-term surges.
Rolling 21-Day Forecasts
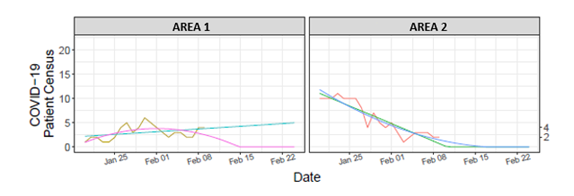
Figure 1: Rolling 21-Day ICU: Lines illustrate actual (jagged), linear (straight), and polynomial (curved) projections. Right y-axis value represents the most recent midnight census in respect to the ending jagged line.
Direct Multi-Step Forecast with Multiple Times Series using XGBoost
The purpose of these forecasts is to supply a one-month projection for ICU and Hospital utilization within relevant Hospitals, specifically for COVID-19 positive patients. The current projections are based on St. Luke’s data and are created using a novel, sophisticated machine learning algorithm. St. Luke’s Advanced Analytics now provides the novel projection for a 30-day horizon.
Direct Multi-Step Forecasting with Multiple Time Series (Direct Forecast) is a methodology that trains on historical data (data already observed and collected) and creates a projection for, in this case, a future date.
Three separate model horizons (1-day, 1-14 day, and 1-30 day) are then used to determine the timespan parameters of the model, which is then fed into the XGBoost portion of the model.
The model currently uses COVID-19 Positive tests per region (Magic Valley and Treasure Valley) as reported by the State of Idaho, Admissions, and Discharges from each Hospital, Idaho State Reopening Phases, and Holidays as predictive features. The addition of policies and discoveries such as the reopening of schools, potential masking policies, or the discovery of a vaccine can be used within the model once the policy or discovery has been enacted and given at least two weeks of training data.
One-Month Forecast: Direct Multi-Step Forecast with Multiple Times Series using XGBoost
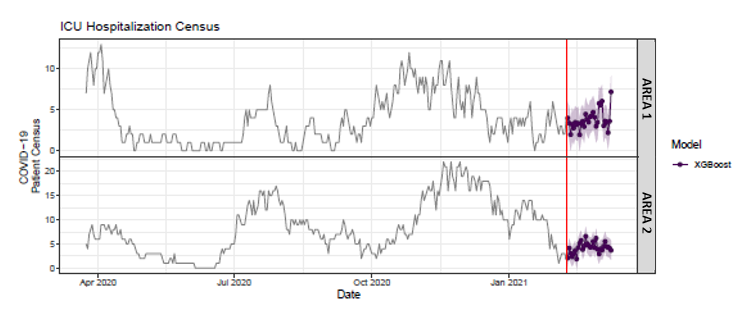
Figure 2: Direct Forecast Projection for ICU Hospitalization Census: Red line indicates the current date and the jagged line before the red line denotes the historical hospitalization census. Purple line after red line is the model’s projection per day with a confidence interval of +/- 2.
What’s Next?
The Advanced Analytics team that developed XGBoost is a finalist for an Aegis Graham Bell Award, an annual award sponsored by the government of India’s Ministry of Electronics and Information Technology. The category St. Luke’s is in is “Combat COVID-19 With Artificial Intelligence.”
St. Luke’s Health System is one of a very select few organizations in healthcare both has the capability and experience in utilizing advanced Machine Learning. We are very proud and excited to serve our communities with the support of cutting-edge technologies.