Real-world considerations for leveraging AI in Healthcare
By Marcos Salganicoff, Ph.D., Director, Data & Analytics, KPMG & Bharat Rao, Ph.D. Principal, Data & Analytics for HealthCare & Life Sciences, KPMG
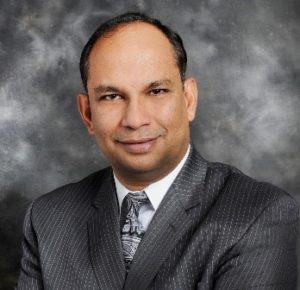
AI’s potential role in healthcare is immense, and ranges from the most advanced personalized medicine algorithms to more mundane, but equally pressing tasks such as robotic-process-automation for tedious and time-consuming clinical documentation, administrative and coding tasks. Additional groundbreaking AI applications in healthcare, including advanced medical image analysis, natural language applications for healthcare automation, and intelligent demand prediction and scheduling, are coming into the mainstream with a tremendous opportunity to improve quality of care, efficiency and patient experience alike.
The healthcare executive faced with the challenges of improving efficiency and quality may view AI as a highly promising cure to what ails them, but successfully leveraging AI in healthcare can be a daunting task for those charged with sorting through the highly-hyped claims.
Through KPMG’s experience in cross-industry and healthcare evaluation, development and deployment of practical AI Solutions by our healthcare industry teams, in combination with our Advanced Data & Analytics Center of Excellence, a set of criteria for evaluating different use cases and related AI technology solutions has been developed. Below are some foundational aspects that should be taken into account when evaluating potential AI-based scenarios and solutions.
Understand what AI Accuracy Statistics Mean to Your Organization
Before purchasing and implementing any AI system, it is important to have a clear set of data available for the system’s accuracy. Often this information is elusive as vendors are hesitant to immediately provide this information. There are a number of well-established accepted technical criteria available to assess accuracy of AI systems (such as false/positive negative rates, receiver-operating curves, precision/recall, etc.) that should be understood in practical terms by those doing the evaluation, and the vendor should provide typical training, test and hold-out statistics, and also be prepared to address variation in these rates within different past deployment sites to show the system is stable. Additionally, you should evaluate system accuracy in light of their impact on your processes, with inputs from key medical, financial and operational stakeholders. For example, a system with a large number of false positives (while having high sensitivity) may be unusable due to a lack of confidence in the system by caregivers, or the high nuisance factor in reviewing each false positive case. In the worst case, it can even result in carrying out unnecessary care in the form of diagnostic procedures or treatments that can possibly lead to additional costs and issues for the patient, if appropriate controls are not put in place to mitigate them.
AI in Healthcare Workflow & Processes
Even the most powerful AI system is ineffective, if it cannot be inserted seamlessly into existing healthcare processes in a convenient and impactful way. It is extremely important to understand how and where the AI system will integrate your clinical, operational and financial workflow & processes. Planning for this demands significant process re-engineering and requires training to realize its benefits. User acceptance of the AI system by key medical, financial and operational stakeholders is also an important consideration for the various stakeholders leveraging AI solutions. In particular, clarity and explanatory capability for the basis of recommendations given by the AI decision support system and its associated predictions are often key to their being accepted and truly successful.
AI and Patient Data in the Cloud
State-of-the-art AI and machine learning systems are increasingly available only within cloud-based platforms and AI-ecosystems such as Microsoft’s Azure, Google and Amazon. In many cases your patient’s health data must meet the AI algorithms in the cloud. Extreme care must be taken to choose a cloud platform and purpose-built environment (IaaS or PaaS) that is engineered and verified as HIPAA or GDPR compliant (as applicable) and designed with risk and cybersecurity measures in mind in order to get access to the latest AI technologies.
Data Use and Governance
As AI systems are always hungry for patient data, it is important to have a strong governance framework in place to ensure those data use agreements with third parties, and appropriate consents are in place with patients providing the data necessary to train and validate the system. Most HIPAA agreements and BAAs (Business Associate Agreements) should cover the use of data for performance/process improvement, but it is important to review these agreements in detail. Terms need to ensure the data can be properly leveraged to train and enhance the AI system, and that clear third-party language is in place in case the vendor desires to retain data for product improvement purposes.
AI Algorithm Risk Management
As AI systems become more integral to the enterprise operations and success, they will carry progressively more risk – both medically and financially. A comprehensive analysis involving medical, legal and financial experts, before deploying an AI system is important step, in terms of understanding and clarifying who ultimately bears the financial and medical risk. Putting a well thought out and vetted set of risk mitigations in place is essential to characterize AI model-risk, and creating and implementing appropriate controls. Risk management should not be an afterthought in the implementation of an AI system.
Conclusion
The above considerations provide a sound starting point for evaluation and decision making to help ensure a successful experience in the use of promising AI solutions in your healthcare enterprise. An experienced and trusted external healthcare AI advisor can be a strong ally in helping you systematically identify and evaluate these many considerations.