Health Data Cube – Implementation of a data pre-aggregation platform for improving information availability
By Dr. Omid Shabestari, Director of Health Analytics & Shea Jessee, Team Lead, Carilion Clinic
What is the average number of medication orders per inpatient visit? Where are the highest ratios occurring? Is this unique to certain medications?
Which providers in your organization consistently rank highest and lowest inpatient satisfaction? What are the particular events or behaviors that lead to these rankings?
How does your organization provide the data needed to answer these questions? Is it accessible to analysts without submitting a request to your reporting team? Can it be presented daily with data as recent as the previous day, or is it only available as an outdated monthly report? These are examples of the data that Carilion Clinic has been able to build into a data cube, developed in the Health Analytics department.
A cube is typically built on top of a data warehouse, where data from various sources has been pulled onto a shared platform.
The cube has been built on top of the organization’s EMR data. It is also connected to other data sources, such as patient satisfaction survey data and information provided by our care quality vendor. The cube has made data available to the organization that was previously accessible only through reporting tools. This bypasses the limitations of computing resources for data analysis as the information is refreshed and recalculated overnight and made available to users the next day.
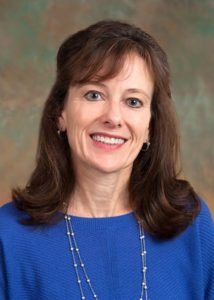
A cube is a model of data represented by logical units called dimensions. It provides aggregated amounts, such as sums, counts, and averages for each element in the dimension. A simple example, using EMR data, would be a patient dimension containing attributes that characterize your patients, such as age, gender, ethnicity, address zip code. For the patient dimension, the data that can be aggregated are information elements such as patient counts, average, maximum and minimum age. Once calculated, they can be sliced by the attributes of other dimensions in the cube. An example would be to see the patient count, broken down by patient age for a particular diagnosis. A cube can also be used to generate commonly used calculations and ratios, such as the change in values over time, and the comparison of actual results to targets using KPI’s. These consistent and transparent calculations facilitate homogeneity of measurements across the organization. In addition, cubes provide row-level security. This facilitates the creation of smart dashboards that will show the information pertaining to the logged-in user without complex programming or need for similar dashboards for different users on the data visualization platform.
A cube is typically built on top of a data warehouse, where data from various sources has been pulled onto a shared platform. The data tables are often built using a dimensional model, where a dimension and fact table are built for each entity. Using this table structure not only facilitates passing data to a cube but also standardizes the data from various data sources and facilitates connections between them. This is especially important when you have conflicting sources of information for the same entity. By using this common table structure and joining tables from different data sources, the data can be sliced by relevant dimensions, which will support data analysis across the organization. For example, the EMR data joined to the patient satisfaction survey data allows analysis of the data by department, diagnosis, and procedures, in addition to any other relevant dimensions.
At Carilion Clinic the data cube is processed nightly so that the data available for analysis is no more than 24 hours old. Several analysis tools can be used with the cube data, including spreadsheets and data visualization tools. The turn-around time for using EMR data to satisfy a report request or analysis has gone from a period of several months to a simple matter of connecting to the cube and building a spreadsheet or dashboard.
Several organizational changes had to be put into place to support the development of the data warehouse and the cube, and to establish them as the single source of truth within the organization. These changes included the establishment of a data governance council and a designated group of data stewards. These groups will standardize definitions of terms and calculations of measures, and help the organization to gain control over, and assure the validity of data.
Creation of an enterprise data warehouse, and building a cube, takes significant effort and buy-in from the organization, but the payoff can be enormous in terms of controlling, validating and providing valuable data across the entire organization. There are many software products available to support cube development. It should be chosen to align with the end-user products that are desired, such as BI and visualization tools. At Carilion, the Health Analytics team will continue to build upon the existing models, bringing in additional data sources providing insight into clinical, financial and operations initiatives. Our goal is uncovering problems and responding with solutions to improve care and reduce cost.