Does your approach to data and analytics undermine Diversity, Inclusion and Equity?
By Heidi Baskfield, VP Population Health & Advocacy, Ellen Sublett, Quality Improvement Specialist, Sana Yousuf, Software Engineer, Children’s Hospital Colorado
Developing an effective work that accomplishes diversity, equity, and inclusion (DEI) within an organization, requires deliberate and consistent intention to restructure everything from recruitment and hiring practices to key components of the healthcare delivery. However, using data and analytics to support DEI work is often overlooked and too often we find ourselves behind on using data equitably. With more healthcare systems voicing a commitment to eradicate racism and support a diverse, equitable, and inclusive culture, data and analytics is the secret sleeper that can either ensure success or result in more of the status quo.
Healthcare systems need to develop a data equity practice to serve as a foundational component to DEI efforts.
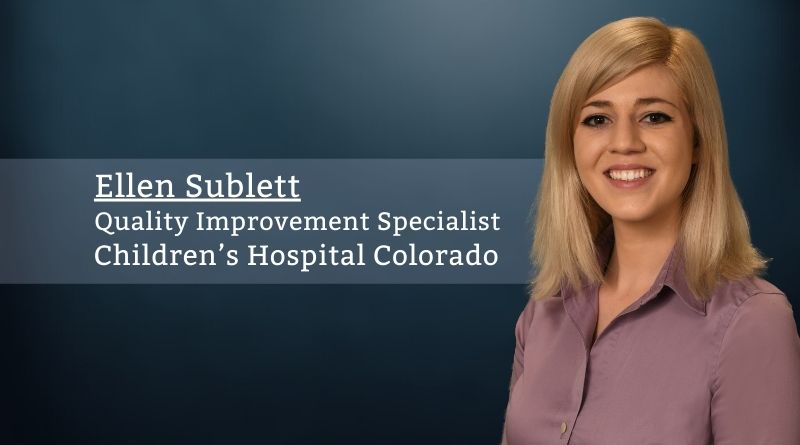
Data is a powerful tool and critical in the world of healthcare. Given this inherent power, there are several potentially negative consequences to how data are used that reinforce systemic inequities rather than reducing them. That is why healthcare systems need to place as much attention and energy into developing data equity strategies as they do in creating equitable human resource or patient care strategies.
Healthcare systems need to develop a data equity practice to serve as a foundational component to DEI efforts. This means understanding who collects data, how data collection processes are designed, how impacted populations are involved in the data collection, and how data is communicated—including any inherent bias in these approaches—is critical.
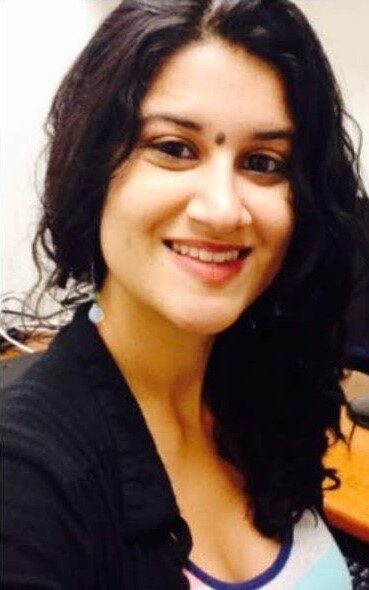
Data equity practices need to ensure that data are continually explored and inequities are highlighted at the individual- and structural-level. These practices should include examining various demographic indicators, promoting rigorous statistical testing, and ensuring that false causal relationships are not readily inferred.
Health systems need to ensure they analyze the appropriate indicators. Structural indicators can be used to reform systemic changes, while individual-level indicators can help us gain insights into a community but are often more susceptible to bias and prejudice interpretation. While these subjective outcomes are often unintentional, they standardize negative systems thinking which only helps re-enforce biases in analysis and interpretation. The value of a data equity practice is that it would create an official process to ensure that where individual-level data must be used, it does not attempt to assume the general population’s experience.
When it comes to the role of statistical testing, it is important to consult with analytic and public health experts to ensure the appropriate tests and indicators are used in the analysis. It is key to exercise caution when conducting statistical tests to avoid masking any observed differences or making false generalizations. Examples of differences that could be impactful can include historical trauma and cultural differences.
A strong equity practice ensures that qualitative and quantitative aspects of data are tied together to provide a rich understanding of populations of interest. Key informant interviews, focus group discussions, and open-ended questions can help frame the data analysis needed to verify the quantitative assessment elements. Often when summarizing qualitative data into common themes, a team member may be subject to internal biases. By having additional and diverse team members review the initial coding of the data, the team gains consensus on common themes from multiple perspectives. With an even more diverse set of eyes combing through the data, we can gain insights into other trends that exist. These trends become important when thinking of the systems of change that need to happen.
There should also be a commitment to sharing the data with communities affected by challenges or topics highlighted in the data so that communities can be part of the analysis, reporting, and ownership of findings. This not only allows the community to have an opportunity to respond to the data, but can lead to new insights, underlying context, and knowledge on the data that you may not have as a person not impacted by the topics of the data.
When presenting data in an equitable manner, it is vital to provide context to the audience. Context can include historical background on the analysis and purpose of the overall project, acknowledging bias and data limitations, and how community voice input was obtained. Historical background can help change the data lens for the audience to be more focused on systematic or structural issues even when the data is at the individual- or group- level. If findings focus on a specific community, be sure to describe how the findings may or may not be generalizable to other communities.
The DEI journey is different for all, but healthcare cannot eradicate racism within our organizations and communities if our approach to data is used to re-enforce it. It will take time and commitment, but we must start now so that we are prepared, rather than delayed, in understanding the impacts of and solutions to addressing health equitably across our patient populations and community.